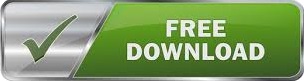
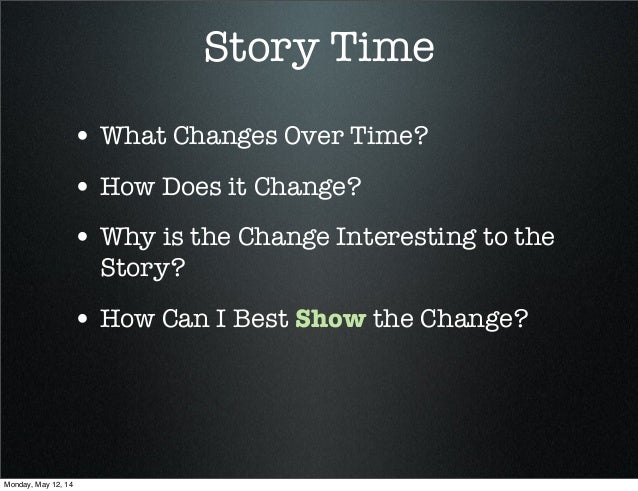

Every year, we have freshmen who have a less-than-stellar first semester. We wanted to have a meeting with these students to help them understand that their first round of classes has put them off-track for graduation. About four years ago, came to me with a problem. I work in a K-12 school in Illinois (about 90 mins from Milwaukee.your cold January viz resonated in my bones!), and this is a revised project we are about to deploy in the coming weeks. We hope you enjoy the various views of variability in data that follow! Until then, check out the #SWDchallenge page for the archives of previous months' challenges and submissions. The next monthly challenge will be announced on March 1st. png) to and we'll work to include any late entries this week (just a reminder that tweeting on its own isn't enough-we don't have time to scrape Twitter for entries, so emailing is the sure way to get your creations included). If you tweeted or thought you submitted one but but don't see it here, email your submission (including your graph attached as. To everyone who submitted examples: THANK YOU for taking the time to create and share your work! The makeovers are posted below in alphabetical order. Like Cole, many people are over cold temperatures! Johannie showed variance in January’s daily average temperature in Quebec, Joshua displayed the average rainfall across time in Portland and Lora visualized the spread between min & max in fall & winter with dots. Weather was another hot topic (pun intended). Ben X had the recent NBA All-Star game on his mind with his visualization of each NBA team’s scoring data (and thoughtful use of color within a spaghetti graph). RJ also tested a new tool: sharing an image of variability in data from his recently released book! (Stay tuned for the next SWD podcast, where he and Cole discuss.)įrom a topics perspective, February means love is in the air… or is it? Several readers showed that, well, it varies… by generation ( Declan’s dating preferences and Lisa’s Valentine’s Day celebrations) and across states ( Kate’s martial rates). Dennis carried over last month’s challenge by trying out a new feature (bump chart) in his tool (PowerBI).

Tools employed included Tableau, Excel, R, JMP, Python and more. Ben J showed examples of numerous ways to visually encode the range of MLB strikeouts-including reference bands and circle histograms. Haley utilized boxplots to visualize the variability of The Simpsons ratings (both within a season and over time) and Meagan chose violin plots to show the variability of Denver’s daily temperature.
#STORYTELLING WITH DATA MOVIE#
Alex gave insight on how certain movie genre’s popularity has varied over time with an area chart to visualize deviation from average. When it comes to chart types, Adina used histograms to show the differing graduation rates between school sizes. This month’s challenge was to visualize variability in data and nearly four dozen delivered! It’s neat to see the variation across submissions, with people making use of numerous chart types, topics, and tools.
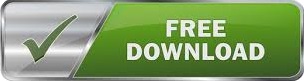